基于PyTorch,如何构建一个简单的神经网络
建立神经网络
import os
import torch
from torch import nn
from torch.utils.data import DataLoader
from torchvision import datasets, transforms
加载训练设备
device = 'cuda' if torch.cuda.is_available() else 'cpu' #检测gpu是否可用,不可用使用cpu
print('Using {} device'.format(device)) #输出使用设备类型
定义类
class NeuralNetwork(nn.Module):
def __init__(self): #定义网络结构
super(NeuralNetwork, self).__init__()
self.flatten = nn.Flatten()
self.linear_relu_stack = nn.Sequential(
nn.Linear(28*28, 512),
nn.ReLU(),
nn.Linear(512, 512),
nn.ReLU(),
nn.Linear(512, 10),
nn.ReLU()
)
def forward(self, x): #前向传播
x = self.flatten(x)
logits = self.linear_relu_stack(x)
return logits
model = NeuralNetwork().to(device) #实例化模型
print(model)
X = torch.rand(1, 28, 28, device=device) #生成(1,28,28)的数据
logits = model(X) #向模型输入数据
pred_probab = nn.Softmax(dim=1)(logits) #调用softmax 将预测值映射为(0,1)间的概率
y_pred = pred_probab.argmax(1) #最大概率对应分类
print(f"Predicted class: {y_pred}")
神经网络各层说明
input_image = torch.rand(3,28,28) #生成(3,28,28)的数据
print(input_image.size())
nn.Flatten 层
flatten = nn.Flatten()
flat_image = flatten(input_image) #(3,28,28)转换为(3,784)
print(flat_image.size())
nn.Linear 层
layer1 = nn.Linear(in_features=28*28, out_features=20) #输入(3,28*28) 输出(3,20)
hidden1 = layer1(flat_image)
print(hidden1.size())
nn.ReLU 层
print(f"Before ReLU: {hidden1}\n\n")
hidden1 = nn.ReLU()(hidden1)
print(f"After ReLU: {hidden1}")
nn.Sequential 层
softmax = nn.Softmax(dim=1)
pred_probab = softmax(logits)
输出模型结构
print("Model structure: ", model, "\n\n")
for name, param in model.named_parameters():
print(f"Layer: {name} | Size: {param.size()} | Values : {param[:2]} \n")
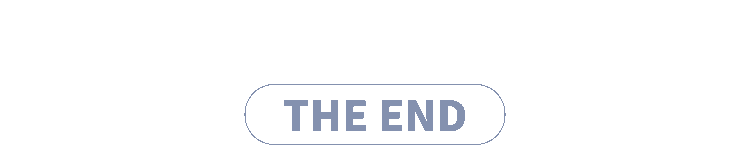
关注公众号:拾黑(shiheibook)了解更多
[广告]赞助链接:
四季很好,只要有你,文娱排行榜:https://www.yaopaiming.com/
让资讯触达的更精准有趣:https://www.0xu.cn/
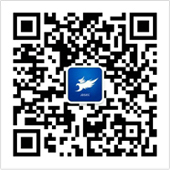
随时掌握互联网精彩
赞助链接
排名
热点
搜索指数
- 1 人生能得几次搏 4948452
- 2 中国00后拿下巴黎奥运首金 4940038
- 3 上海航空回应为等外籍人士致航班延误 4857574
- 4 中国军团巴黎奥运图鉴来了 4766830
- 5 女子双人3米板跳水决赛 4639821
- 6 中国奥运军团首日开门红 4572050
- 7 租客退租将小金毛遗弃在公寓近一个月 4471027
- 8 南京工业大学35名研究生被退学处理 4394682
- 9 女子被捆绑后扔山洞?假的 4223008
- 10 切尔西名宿德罗巴为贾玲撑伞 4110505